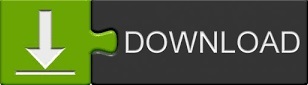
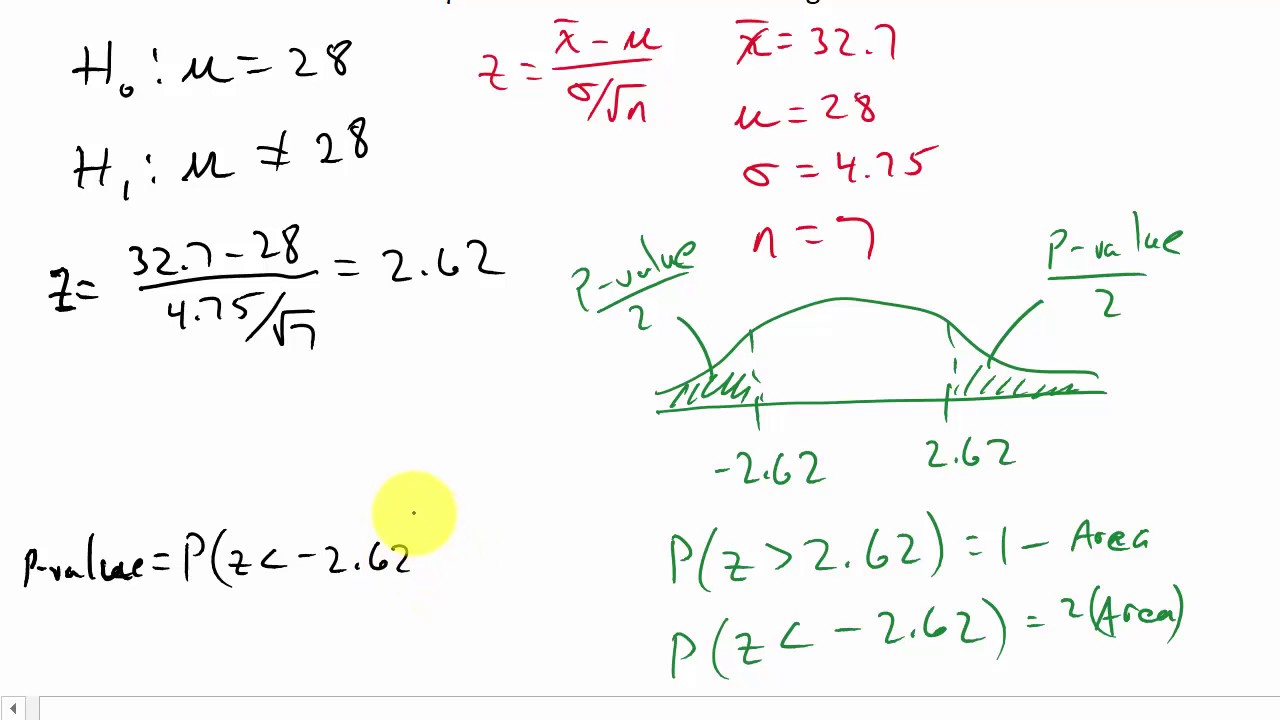
As you can see in the table above, the significance level is set at 0.05. Significance levels are usually set at 0.05, 0.10 or 0.15. The significance level is the chance of committing a type 1 error, which is when we reject a null hypothesis when it is actually true. In order to figure out whether or not we will accept a hypothesis or not, we first need to set a significance level for our hypothesis test. To test this hypothesis, we use the probability distribution of salaries to see how likely a mean salary of below 10,000 would be.

However, our proposed explanation might not be true. Here, the null hypothesis states that the salary of a group of people is below 10,000. The mean salary of a group is more than 10,000 Let’s take the following null and alternative hypotheses as an example.
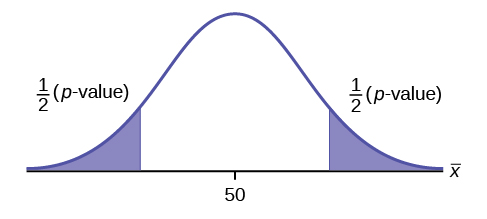
This is what makes them so useful in hypothesis testing.īecause probability distributions can be used in hypothesis testing, we can determine how likely a certain value is to occur or not. However, because probability distributions display the probabilities of each value, they can be used to predict how likely certain values are. In other words, both can give us a picture of the mean and standard deviation. Lower than it would normally be, low values “deflate” the meanīoth the frequency and probability distributions can tell us about a variable’s spread and centre. Values are centred around higher values with only some values on the lower end The difference between the two distributions can be seen in the table below. These distributions are skewed distributions, which means that the majority of the values centre around one end of the range of values. For example, the frequency distribution can tell us whether the variable is skewed or not. The major difference, however, is what they tell us about the data. The probability of a person being a given age from 1-100Īs you can see, both types of distributions give us information about how the data looks like. The number or people in each age group from 1-100ĭisplays the probability of each value of a variable occurring In order to understand the difference between the two, check out their definitions summarized in the table below.ĭisplays the frequency of each value of a variable occurring There are two main things we can mean when we refer to a distribution: a frequency distribution and a probability distribution. In order to understand what type of hypothesis test you need to run, you need to first determine the probability distribution of your variable.

When the test statistic is outside the region of rejection When the test statistic is inside the region of rejection In statistical hypothesis testing, there are two types of hypotheses, summarized below. Inferential statistics try to make predictions based off of a sample dataset. Take a look at the image below, describing the definition of inferential statistics. Statistical hypothesis testing, on the other hand, is one of the methods involved in inferential statistics.
#Two tailed hypothesis test calculator software#
This can be through measurements, using models, software and more. Hypothesis testing, in general, is very simple: it means testing a hypothesis. The mean number of votes is different between two populations: one with no media influence, one with media influence Votes increase with increased media pressure Take a look at the table below, comparing the general definition of a hypothesis with that of a statistical hypothesis.Īn assumption about a parameter that can be tested with a data set Hypotheses in statistics can help us suggest explanations for probabilistic events. While hypotheses are used in many areas, such as psychology or biology, they can also be used in statistics. In fact, you have probably made a hypothesis today! More rain leads to less tourists in the cityĮxtreme temperatures lead to lower battery lifeĪn increase in air quality leads to a decrease in diseasesĪs you can see, hypotheses are quite simple. It’s that simple! Take a look at the table below to get some real life examples of hypotheses. Hypothesis Definition A hypothesis is a suggested explanation for some event.
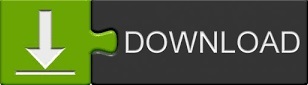